There’s no question that 2020 proved to be a banner year for 3D printing / additive manufacturing (AM). Amidst the disruption caused by the COVID-19 pandemic, the technology firmly cemented its position as a serious alternative to existing production methods. Carlos Zwikker reports.
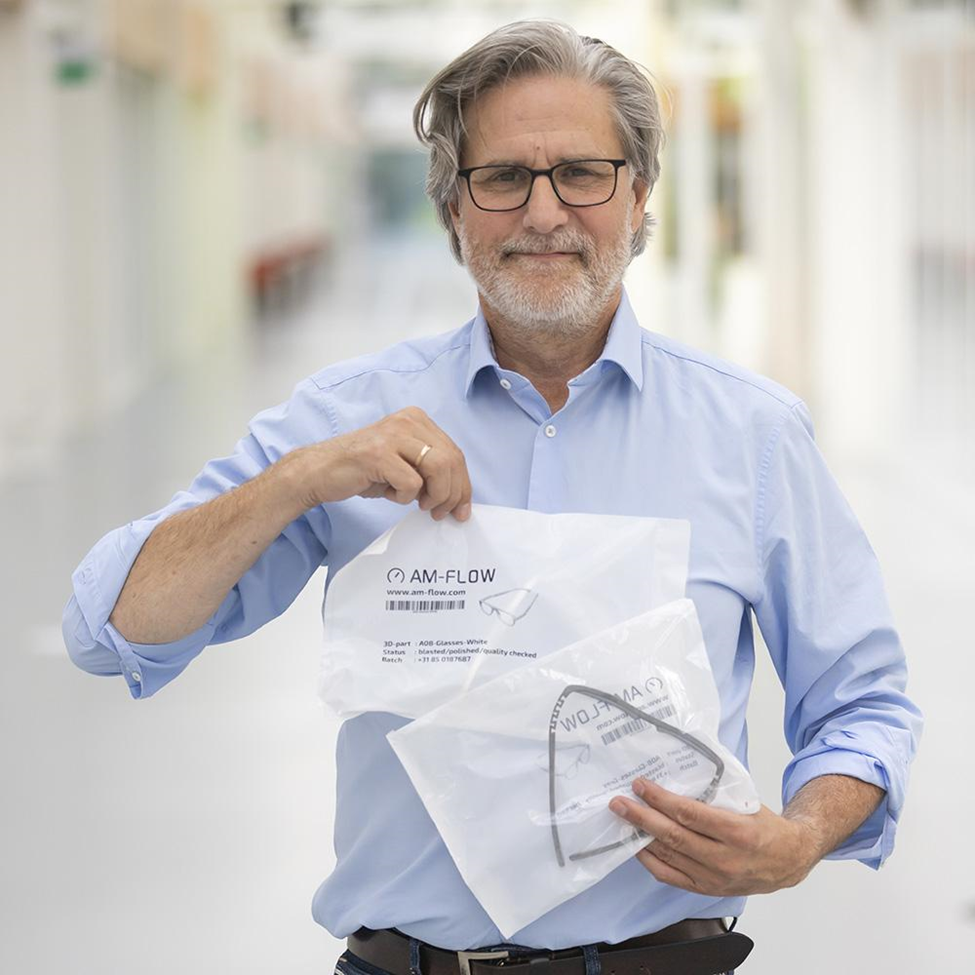
Of course, even before COVID, many companies had embraced the benefits of AM, taking advantage of opportunities around localised production, digital inventories and on-demand manufacturing. However, as the business case for AM continues to stack up, and more and more companies begin to look at how they can scale-up production, it is becoming clear that it is high time to address the elephant in the room – the hugely inefficient post-processing phase.
Think about it: 3D printing is a highly automated process, right up until the point when the printed part leaves the machine. After that, everything else, from picking and sorting to packing, has to be done manually. That typically means that companies must employ a team of people to painstakingly identify and pick each part as it leaves the printer, then physically transport it to the next post-processing station – and possibly several more after that – before ultimately bringing everything back together to bag and send to the customer. That is an incredibly labour-intensive, costly and inefficient model that leaves processes hugely vulnerable to human error.
To try to offset increasing labour costs, we’ve seen some companies outsource production to lower wage countries. However, that completely defeats many of the advantages of using AM. The fact is, if we want to harness the benefits of AM, such as localised on-demand production, reduced carbon footprint, and small batch production, we have to find ways to automate post-processing – and quickly. From a commercial perspective, our benchmark needs to be existing traditional manufacturing technologies. Take injection moulding, for example. It is a mature, fully-automated technology – attributes that make it very efficient. While we don’t necessarily need to compete directly with injection moulding – and we’re definitely never going to replace it – as an industry we need to be competitive from a price-per-part and lead-time perspective.
Adopting a new mindset
A question we often hear asked is why companies are only thinking about post-processing now, especially those that have been using AM for years. The answer is that despite recent advances in the use of 3D printing, it is still a relatively immature industry. Most AM production environments have grown slowly over time, in response to specific challenges or opportunities. Companies have focused on the technology, slowly adding new printers and expanding their use of materials as required, rather than thinking about the production process or workflow. It is only now that AM has proven itself as a viable and useful technology and companies are looking to scale-up their operations that the post-production stage has come under greater scrutiny.
The other reason very few companies have explored opportunities to automate AM post-processing is that, quite simply, the technology has not existed to enable them to do so. It is only with recent advances in machine learning and post-processing hardware and software that full automation of AM post-processing is finally achievable.
New opportunities bring new challenges
Another question we are often asked is one that applies not just to post-processing solutions, but to any type of ‘add on’ system, and that is, ‘will it work with my existing systems?’ As the AM ecosystem continues to expand and become more sophisticated, I believe this question will become increasingly pertinent. In some respects, companies who are only now starting to move into AM have the advantage here, as they can implement a fully-integrated AM workflow – one that encompasses post-processing – from the outset. For companies who already have established AM processes, it is more of a challenge. The last thing they want to do is have to stop production and redesign systems from scratch.
The onus is therefore on solution providers to create systems that are, in effect, ‘open’. Or, in other words, they can be seamlessly integrated with any system currently in use. As I previously mentioned, most AM production environments have grown organically over time to meet specific needs or challenges. As a result, the landscape is extremely fragmented – nothing is standardised and there are a lot of proprietary systems in place. To enable a swift and smooth transition to full automation, it is imperative that any post-processing solution is able to ‘plug in’ to whatever systems are in use. This means it should be compatible with all existing MES or ERP software and can also be easily integrated with external systems such as cleaning and part quality enhancing post-processing hardware.
The AM ecosystem as a whole is at an exciting and pivotal point in the technology’s evolution. In order to unlock its full potential, it is crucial that companies stop thinking about post-processing in isolation – if they are even thinking about it at all – and consider how it can be implemented into a seamless end-to-end AM workflow. It is only by doing so that AM will be able to deliver on its promises off the last decade and ascend to its rightful place as a fully-digital, fully-connected part of the factory of the future.
Application
Putting Words into action
The first step to automating AM post-processing is to be able to identify each and every part as it leaves the 3D printer. As a result, machines are coming to market that are capable of identifying 3D printed parts in a split second. For example, AM-Flow’s AM-VISION identification module is fitted with ten cameras that take multiple images of a printed part as it passes through the unit, which are then cross-referenced with meta-data from its STL file. From this information, the machine can identify parts in as little as 0.2 seconds, regardless of how many parts are being printed or how they are positioned on the conveyor belt. What’s more, by harnessing the power of machine learning, the identification process can be fine-tuned over time, so that it continually becomes more accurate and reliable.
The ability to identify 3D printed parts makes full track and trace capability a reality, which is another important milestone that must be achieved to bring the efficiency of AM into line with traditional manufacturing processes. Of course, once you can automatically identify a 3D printed part, you can also sort it, pick it, transport it and bag it. As a result, we are also seeing the arrival of modules to automate each of these individual processes as well, as part of a seamless, end-to-end digitalised workflow.